Niall Palfreyman wrote this on the board to introduce a course in differential equations:
- The Secret of the Universe in 6 sentences
- Nature always integrates flows over time
- Flows always differentiate fields over space
- Structure determines behaviour
- Algebra is the study of structure
- Dynamics is the study of behaviour
I like it.
A little explanation is in order. I have my morning coffee in hand. It’s warmer than the room, so it’s cooling off. It’s heat winds up in the room. If I want to manage my coffee well, neither burning my tongue nor gagging down cold sludge, I need to be able to make some predictions about the future behavior of my cuppa joe. I won’t get far by postulating demons randomly stealing calorics from my cup, though that might provide a soothingly fatalistic outlook. I’m much better off if I understand how and why coffee cools.
#2, the “nature integrates flows” part of the system looks like this:

Each box represents an accumulation of heat (that’s the integral). Each pipe represents a flow of heat from one place to another. The heat currently in the house is simply the net result of all the inflows from coffee cups, and all the losses to the outside world, over all time (of course, there are other flows to consider, like my computers warming the room, and losses to the snowy outside).
In the same way, the number of people in a room is the net accumulation of all the people who ever entered, less all those who ever left. A neat thing about this is that the current heat in the cup, or count of people in a room, is a complete description of the state of the system. You don’t need to know the detailed history of inflows and outflows, because you can simply take the temperature of the cup or count the people in the room to measure the accumulated effects of all the past events.
The next question is, why does the heat flow? That’s what #3 is about. Heat follows temperature gradients, as water flows downhill. Here’s a temperature field for a coffee cup:
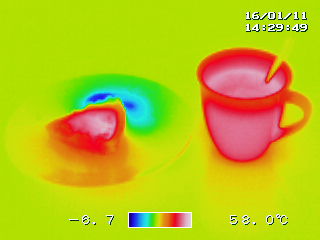
wikimedia commons
Heat will flow from the hot (red) cup into the cool (green) environment. The flow will be fastest where the gradient is steepest – i.e. where there’s the greatest temperature difference over a unit of space. That’s the “flows differentiate fields” part. Other properties also matter, like the thermal conductivity of the cup, air currents in the room, insulation in the wall, and heat capacity of coffee, and these can also be described as distributions over space or fields. That adds the blue to the model above:
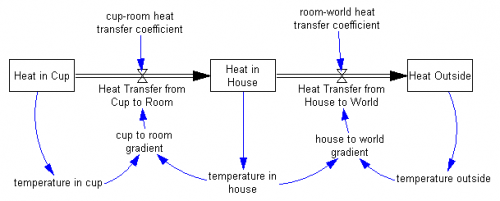
The blue arrows describe why the flows flow. These are algebraic expressions, like Heat Transfer from Cup to Room = Cup to Room Gradient/Cup-Room Heat Transfer Coefficient. They describe the structure – the “why” – of the system (#5).
The behavior of the system, i.e. how fast my coffee cools, is determined by the structure described above (#4). If you change the structure, by using an insulated mug to change the cup-room heat transfer coefficient for example, you change the behavior – the coffee cools more slowly.* The search for understanding about coffee cups, nuclear reactors, and climate is essentially an effort to identify structures that explain the dynamics or patterns of behavior that we observe in the world.
* Update: added a sentence for clarification, and corrected numbering.