Does DNA IQ testing create a meritocracy, or merely reinforce existing biases?
Technology Review covers new efforts to use associations between DNA and IQ.
… Intelligence is highly heritable and predicts important educational, occupational and health outcomes better than any other trait. Recent genome-wide association studies have successfully identified inherited genome sequence differences that account for 20% of the 50% heritability of intelligence. These findings open new avenues for research into the causes and consequences of intelligence using genome-wide polygenic scores that aggregate the effects of thousands of genetic variants.
The new genetics of intelligence
Robert Plomin and Sophie von Stumm
I have no doubt that there’s much to be learned here. However, research is not all they’re proposing:
IQ GPSs will be used to predict individuals’ genetic propensity to learn, reason and solve problems, not only in research but also in society, as direct-to-consumer genomic services provide GPS information that goes beyond single-gene and ancestry information. We predict that IQ GPSs will become routinely available from direct-to-consumer companies along with hundreds of other medical and psychological GPSs that can be extracted from genome-wide genotyping on SNP chips. The use of GPSs to predict individuals’ genetic propensities requires clear warnings about the probabilistic nature of these predictions and the limitations of their effect sizes (BOX 7).
Although simple curiosity will drive consumers’ interests, GPSs for intelligence are more than idle fortune telling. Because intelligence is one of the best predictors of educational and occupational outcomes, IQ GPSs will be used for prediction from early in life before intelligence or educational achievement can be assessed. In the school years, IQ GPSs could be used to assess discrepancies between GPSs and educational achievement (that is, GPS-based overachievement and underachievement). The reliability, stability and lack of bias of GPSs make them ideal for prediction, which is essential for the prevention of problems before they occur. A ‘precision education’ based on GPSs could be used to customize education, analogous to ‘precision medicine’
There are two ways “precision education” might be implemented. An egalitarian model would use information from DNA IQ measurements to customize resource allocations, so that all students could perform up to some common standard:
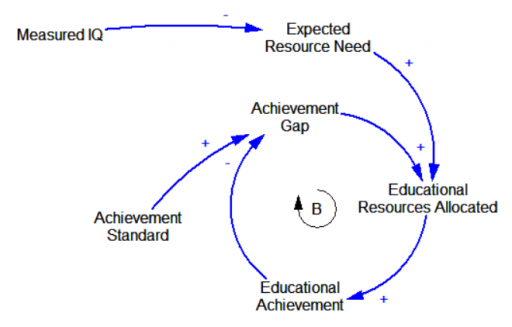
An efficiency model, by contrast, would use IQ measurements to set achievement expectations for each student, and customize resources to ensure that students who are underperforming relative to their DNA get a boost:
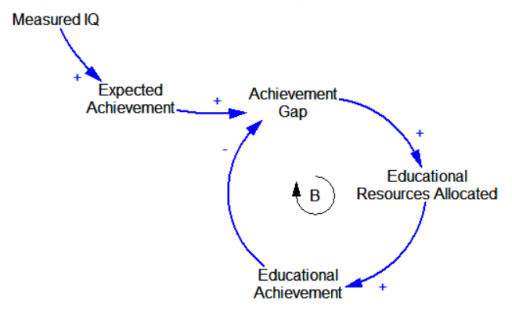
This latter approach is essentially a form of tracking, in which DNA is used to get an early read on who’s destined to flip bonds, and who’s destined to flip burgers.
One problem with this scheme is noise (as the authors note, seemingly contradicting their own abstract’s claim of reliability and stability). Consider the effect of a student receiving a spuriously low DNA IQ score. Under the egalitarian scheme, they receive more educational resources (enabling them to overperform), while under the efficiency scheme, resources would be lowered, leading self-fulfillment of the predicted low performance. The authors seem to regard this as benign and self-correcting:
By contrast, GPSs are ‘less dangerous’ because they are intrinsically probabilistic, not hardwired and deterministic like single-gene disorders. It is important to recall here that although all complex traits are heritable, none is 100% heritable. A similar logic can be applied to IQ scores: although they have great predictive validity for key life outcomes, IQ is not deterministic but probabilistic. In short, an individual is always more than the sum of their genes or their IQ scores.
I think this might be true when you consider the local effects on the negative loops governing resource allocation. But I don’t think that remains true when you put it in context. Education is a nest of positive feedbacks. This creates path dependence that amplifies errors in resource allocation, whether they come from subjective teacher impressions or DNA measurements.
In a perfect world, DNA-IQ provides an independent measurement that’s free of those positive feedbacks. In that sense, it’s perfectly meritocratic:
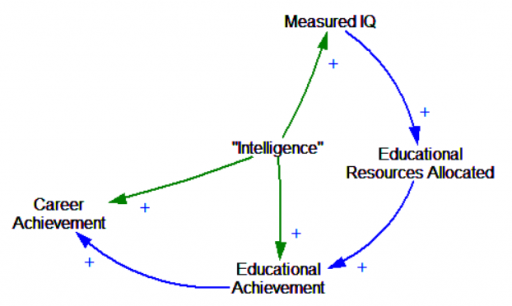
But how do you decide what to measure? Are the measurements good, or just another way to institutionalize bias? This is hotly contested. Let’s suppose that problems of gender and race/ethnicity bias have been, or can be solved. There are still questions about what measurements correlate with better individual or societal outcomes. At some point, implicit or explicit choices have to be made, and these are not value-free. They create reinforcing feedbacks:
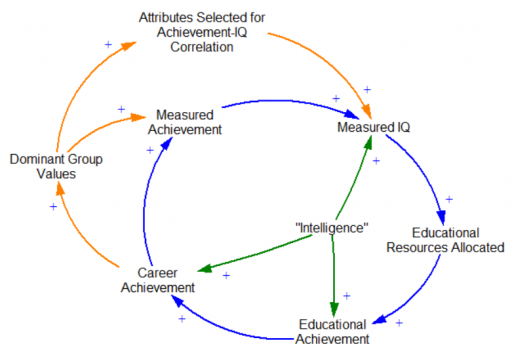
I think it’s inevitable that, like any other instrument, DNA IQ scores are going to reflect the interests of dominant groups in society. (At a minimum, I’d be willing to bet that IQ tests don’t measure things that would result in low scores for IQ test designers.) If that means more Einsteins, Bachs and Ghandis, maybe it’s OK. But I don’t think that’s guaranteed to lead to a good outcome. First, there’s no guarantee that a society composed of apparently high-performing individuals is in itself high-performing. Second, the dominant group may be dominant, not by virtue of faster CPUs in their heads, but something less appetizing.
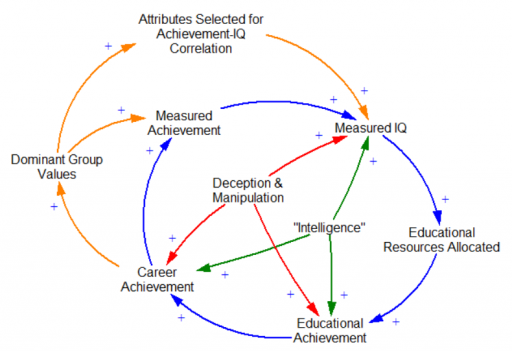
I think there’s no guarantee that DNA IQ will not reflect attributes that are dysfunctional for society. We would hate to inadvertently produce more Stalins and Mengeles by virtue of inadvertent correlations with high achievement of less virtuous origin. And certainly, like any instrument used for high-stakes decisions, the pressure to distort and manipulate results will increase with use.
Note that if education is really egalitarian, the link between Measured IQ and Educational Resources Allocated reverses polarity, becoming negative. Then the positive loops become negative loops, and a lot of these problems go away. But that’s not often a choice societies make, presumably because egalitarian education is in itself contrary to the interests of dominant groups.
I understand researchers’ optimism for this technology in the long run. But for now, I remain wary, due to the decided lack of systems thinking about the possible side effects. In similar circumstances, society has made poor choices about teacher value added modeling, easily negating any benefits it might have had. I’m expecting a similar outcome here.