Or, Friends don’t let friends work for hire.
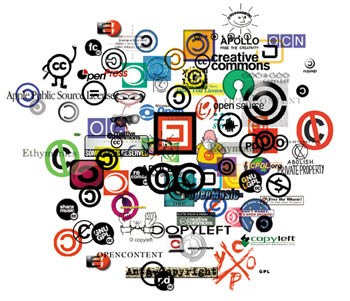
Image Copyright 2004 Lawrence Liang, Piet Zwart Institute, licensed under a Creative Commons License
Photographers and other media workers hate work for hire, because it’s often a bad economic tradeoff, giving up future income potential for work that’s underpaid in the first place. But at least when you give up rights to a photo, that’s the end of it. You can take future photos without worrying about past ones.
For models and software, that’s not the case, and therefore work for hire makes modelers a danger to themselves and to future clients. The problem is that models draw on a constrained space of possible formulations of a concept, and tend to incorporate a lot of prior art. Most of the author’s prior art is probably, in turn, things learned from other modelers. But when a modeler reuses a bit of structure – say, a particular representation of a supply chain or a consumer choice decision – under a work for hire agreement, title to those equations becomes clouded, because the work-for-hire client owns the new work, and it’s hard to distinguish new from old.
The next time you reuse components that have been used for work-for-hire, the previous client can sue for infringement, threatening both you and future clients. It doesn’t matter if the claim is legitimate; the lawsuit could be debilitating, even if you could ultimately win. Clients are often much bigger, with deeper legal pockets, than freelance modelers. You also can’t rely on a friendly working relationship, because bad things can happen in spite of good intentions: a hostile party might acquire copyright through a bankruptcy, for example.
The only viable approach, in the long run, is to retain copyright to your own stuff, and grant clients all the license they need to use, reproduce, produce derivatives, or whatever. You can relicense a snippet of code as often as you want, so no client is ever threatened by another client’s rights or your past agreements.
Things are a little tougher when you want to collaborate with multiple parties. One apparent option, joint ownership of copyright to the model, is conceptually nice but actually not such a hot idea. First, there’s legal doctrine to the effect that individual owners have a responsibility not to devalue joint property, which is a problem if one owner subsequently wants to license or give away the model. Second, in some countries, joint owners have special responsibilities, so it’s hard to write a joint ownership contract that works worldwide.
Again, a viable approach is cross-licensing, where creators retain ownership of their own contributions, and license contributions to their partners. That’s essentially the approach we’ve taken within the C-ROADS team.
One thing to avoid at all costs is agreements that require equation-level tracking of ownership. It’s fairly easy to identify individual contributions to software code, because people tend to work in containers, contributing classes, functions or libraries that are naturally modular. Models, by contrast, tend to be fairly flat and tightly interconnected, so contributions can be widely scattered and difficult to attribute.
Part of the reason this is such a big problem is that we now have too much copyright protection, and it lasts way too long. That makes it hard for copyright agreements to recognize where we see far because we stand on the shoulders of giants, and distorts the balance of incentives intended by the framers of the constitution.
In the academic world, model copyright issues have historically been ignored for the most part. That’s good, because copyright is a hindrance to progress (as long as there are other incentives to create knowledge). That’s also bad, because it means that there are a lot of models out there that have not been placed in the public domain, but which are treated as if they were. If people start asserting their copyrights to those, things could get messy in the future.
A solution to all of this could be open source or free software. Copyleft licenses like the GPL and permissive licenses like Apache facilitate collaboration and reuse of models. That would enable the field to move faster as a whole through open extension of prior work. C-ROADS and C-LEARN and component models are going out under an open license, and I hope to do more such experiments in the future.
Update: I’ve posted some examples.